15 Sep 2023
New Artificial Intelligence project tackles intractable engineering problems to assist the journey to Net Zero
The project uses machine learning methods and practical digital twins to revolutionise the optimisation of a range of energy and transport systems and support the UK’s journey to net zero targets
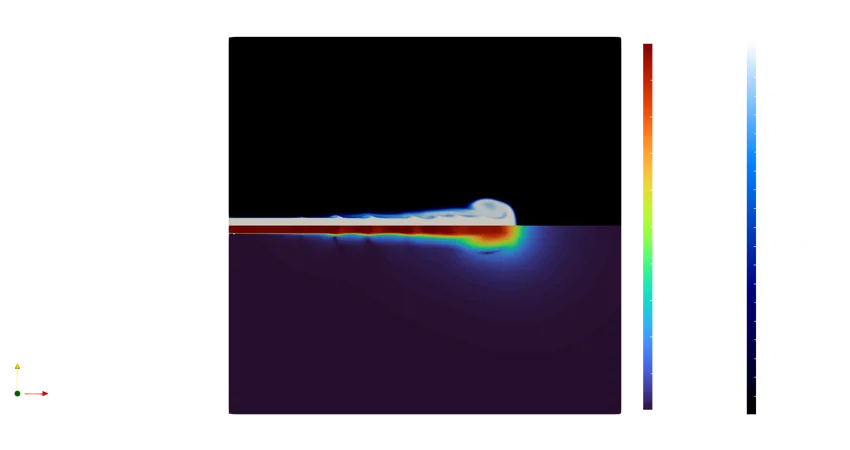
Simulation of Hydrogen Jet Formation After Accidental Release. The simulations were performed by Dr Giovanni Tretola who works as post doc in the project
The research vision is to create a multi-disciplinary framework and toolbox to seamlessly combine high-performance computing and machine learning, utilising real-time digital twins and energy-efficient AI algorithms. The societal vision is to undertake engagement and dissemination activities across industries, the government, and policymakers, combining technical research and innovation through evidence-based examples for energy and transport optimisation.
We are delighted to collaborate with such a talented consortium of leading researchers, towards bringing a step change in net zero pathway by leveraging the amazing breakthroughs in modern techniques of AI.
The project brings together researchers from Imperial, Cambridge, Oxford, and Edinburgh involving various research councils and a diverse range of expertise from across the country. The research aims to build upon existing software and methods previously developed in large UK and EU funded initiatives with the gap between technical methods and policy making being bridged by a dedicated work package and the involvement of two All-Party Parliamentary Groups.
The team in Oxford is led by Associate Professor Konstantina Vogiatzaki as primary investigator and focuses on a work package maximising the reliability, safety, and operational efficiency of hydrogen-based systems. Hydrogen and its carriers such as ammonia have been identified as key fuels to achieving decarbonisation. But to help deliver on the Net Zero sustainability promise, it is crucial to model the interaction between hydrogen reaction chemistry and the environment accurately and efficiently to optimise future systems.
As hydrogen experiments require specialist cryogenic storage conditions, and the gas is extremely flammable, simulations can prove to be expensive even if they produce high-quality data. Hydrogen datasets are, therefore, sparse, and multi-fidelity. This new project aims to design digital twins with surrogate modelling that extrapolates well outside the experimental data, thus reducing costs and safety concerns.
The work will build upon the achievements of Professor Vogiatzaki’s previous UKRI Innovation Fellowship: She says, “During this project we aspire to use the extensive expertise of our group in modelling complex multiphase flows and cryogenic fluids to support the wider and more safe use of liquid hydrogen through the development of real time digital twins based on CFD and ML. Moreover, the strong industrial participation in the project will allow us to better understand industry needs, and to adapt our numerical tools accordingly to maximise the real-world impact.”